Facial Action Coding System (FACS) is a system to taxonomize human facial movements by their appearance on the face, based on a system originally developed by a Swedish anatomist named Carl-Herman Hjortsjö.[1] It was later adopted by Paul Ekman and Wallace V. Friesen, and published in 1978.[2] Ekman, Friesen, and Joseph C. Hager published a significant update to FACS in 2002.[3] Movements of individual facial muscles are encoded by FACS from slight different instant changes in facial appearance.[4] It is a common standard to systematically categorize the physical expression of emotions, and it has proven useful to psychologists and to animators. Due to subjectivity and time consumption issues, FACS has been established as a computed automated system that detects faces in videos, extracts the geometrical features of the faces, and then produces temporal profiles of each facial movement.[4]
The pioneer F-M Facial Action Coding System 3.0 (F-M FACS 3.0) [5] was created in 2018 by Armindo Freitas-Magalhães, and presents 5,000 segments in 4K, using 3D technology and automatic and real-time recognition (FaceReader 7.1).The F-M FACS 3.0 features 8 pioneering action units (AUs), 22 pioneering tongue movements (TMs), and a pioneering Gross Behavior GB49 (Crying), in addition to functional and structural nomenclature.[6]
F-M NeuroFACS 3.0 is the latest version created in 2019 by Dr. Freitas-Magalhães.[7]
- 2Codes for action units
- 2.3List of action units and action descriptors (with underlying facial muscles)
Uses[edit]
Facial Action Coding System Emily B. Prince, Katherine B. Martin, & Daniel S. Messinger The Facial Action Coding System (FACS) is a widely used protocol for recognizing and labelling facial expression by describing the movement of muscles of the face. FACS is used to. Oct 02, 2019 The Facial Action Coding System (FACS) is a comprehensive, anatomically based system for describing all visually discernible facial movement. It breaks down facial expressions into individual components of muscle movement, called Action Units (AUs).
Using FACS [8] human coders can manually code nearly any anatomically possible facial expression, deconstructing it into the specific action units (AU) and their temporal segments that produced the expression. As AUs are independent of any interpretation, they can be used for any higher order decision making process including recognition of basic emotions, or pre-programmed commands for an ambient intelligent environment. The FACS Manual is over 500 pages in length and provides the AUs, as well as Ekman's interpretation of their meaning.
FACS defines AUs, which are a contraction or relaxation of one or more muscles. It also defines a number of Action Descriptors, which differ from AUs in that the authors of FACS have not specified the muscular basis for the action and have not distinguished specific behaviors as precisely as they have for the AUs.
For example, FACS can be used to distinguish two types of smiles as follows:[9]
- Insincere and voluntary Pan-Am smile: contraction of zygomatic major alone
- Sincere and involuntary Duchenne smile: contraction of zygomatic major and inferior part of orbicularis oculi.
Although the labeling of expressions currently requires trained experts, researchers have had some success in using computers to automatically identify FACS codes, and thus quickly identify emotions.[10]Computer graphical face models, such as CANDIDE or Artnatomy, allow expressions to be artificially posed by setting the desired action units.
The use of FACS has been proposed for use in the analysis of depression,[11] and the measurement of pain in patients unable to express themselves verbally.[12]
FACS is designed to be self-instructional. People can learn the technique from a number of sources including manuals and workshops,[13] and obtain certification through testing.[14] The original FACS has been modified to analyze facial movements in several non-human primates, namely chimpanzees,[15] rhesus macaques,[16] gibbons and siamangs,[17] and orangutans.[18] More recently, it was adapted for a domestic species, the dog.[19]
Thus, FACS can be used to compare facial repertoires across species due to its anatomical basis. A study conducted by Vick and others (2006) suggests that FACS can be modified by taking differences in underlying morphology into account. Such considerations enable a comparison of the homologous facial movements present in humans and chimpanzees, to show that the facial expressions of both species result from extremely notable appearance changes. The development of FACS tools for different species allows the objective and anatomical study of facial expressions in communicative and emotional contexts. Furthermore, a cross-species analysis of facial expressions can help to answer interesting questions, such as which emotions are uniquely human.[20]
EMFACS (Emotional Facial Action Coding System)[21] and FACSAID (Facial Action Coding System Affect Interpretation Dictionary)[22] consider only emotion-related facial actions. Examples of these are:
Emotion | Action units |
---|---|
Happiness | 6+12 |
Sadness | 1+4+15 |
Surprise | 1+2+5B+26 |
Fear | 1+2+4+5+7+20+26 |
Anger | 4+5+7+23 |
Disgust | 9+15+16 |
Contempt | R12A+R14A |
Codes for action units[edit]
For clarification, FACS is an index of facial expressions, but does not actually provide any bio-mechanical information about the degree of muscle activation. Though muscle activation is not part of FACS, the main muscles involved in the facial expression have been added here for the benefit of the reader.
Action units (AUs) are the fundamental actions of individual muscles or groups of muscles.
Action descriptors (ADs) are unitary movements that may involve the actions of several muscle groups (e.g., a forward‐thrusting movement of the jaw). The muscular basis for these actions hasn't been specified and specific behaviors haven't been distinguished as precisely as for the AUs.
For most accurate annotation, FACS suggests agreement from at least two independent certified FACS encoders.
Intensity scoring[edit]
Intensities of FACS are annotated by appending letters A–E (for minimal-maximal intensity) to the action unit number (e.g. AU 1A is the weakest trace of AU 1 and AU 1E is the maximum intensity possible for the individual person).
- A Trace
- B Slight
- C Marked or pronounced
- D Severe or extreme
- E Maximum
Other letter modifiers[edit]
There are other modifiers present in FACS codes for emotional expressions, such as 'R' which represents an action that occurs on the right side of the face and 'L' for actions which occur on the left. An action which is unilateral (occurs on only one side of the face) but has no specific side is indicated with a 'U' and an action which is unilateral but has a stronger side is indicated with an 'A.'
List of action units and action descriptors (with underlying facial muscles)[edit]
Main codes[edit]
AU number | FACS name | Muscular basis |
---|---|---|
0 | Neutral face | |
1 | Inner brow raiser | frontalis (pars medialis) |
2 | Outer brow raiser | frontalis (pars lateralis) |
4 | Brow lowerer | depressor glabellae, depressor supercilii, corrugator supercilii |
5 | Upper lid raiser | levator palpebrae superioris, superior tarsal muscle |
6 | Cheek raiser | orbicularis oculi (pars orbitalis) |
7 | Lid tightener | orbicularis oculi (pars palpebralis) |
8 | Lips toward each other | orbicularis oris |
9 | Nose wrinkler | levator labii superioris alaeque nasi |
10 | Upper lip raiser | levator labii superioris, caput infraorbitalis |
11 | Nasolabial deepener | zygomaticus minor |
12 | Lip corner puller | zygomaticus major |
13 | Sharp lip puller | levator anguli oris (also known as caninus) |
14 | Dimpler | buccinator |
15 | Lip corner depressor | depressor anguli oris (also known as triangularis) |
16 | Lower lip depressor | depressor labii inferioris |
17 | Chin raiser | mentalis |
18 | Lip pucker | incisivii labii superioris and incisivii labii inferioris |
19 | Tongue show | |
20 | Lip stretcher | risorius w/ platysma |
21 | Neck tightener | platysma |
22 | Lip funneler | orbicularis oris |
23 | Lip tightener | orbicularis oris |
24 | Lip pressor | orbicularis oris |
25 | Lips part | depressor labii inferioris, or relaxation of mentalis or orbicularis oris |
26 | Jaw drop | masseter; relaxed temporalis and internal pterygoid |
27 | Mouth stretch | pterygoids, digastric |
28 | Lip suck | orbicularis oris |
Head movement codes[edit]
AU number | FACS name | Action |
---|---|---|
51 | Head turn left | |
52 | Head turn right | |
53 | Head up | |
54 | Head down | |
55 | Head tilt left | |
M55 | Head tilt left | The onset of the symmetrical 14 is immediately preceded or accompanied by a head tilt to the left. |
56 | Head tilt right | |
M56 | Head tilt right | The onset of the symmetrical 14 is immediately preceded or accompanied by a head tilt to the right. |
57 | Head forward | |
M57 | Head thrust forward | The onset of 17+24 is immediately preceded, accompanied, or followed by a head thrust forward. |
58 | Head back | |
M59 | Head shake up and down | The onset of 17+24 is immediately preceded, accompanied, or followed by an up-down head shake (nod). |
M60 | Head shake side to side | The onset of 17+24 is immediately preceded, accompanied, or followed by a side to side head shake. |
M83 | Head upward and to the side | The onset of the symmetrical 14 is immediately preceded or accompanied by a movement of the head, upward and turned and/or tilted to either the left or right. |
Eye movement codes[edit]
AU number | FACS name | Action |
---|---|---|
61 | Eyes turn left | |
M61 | Eyes left | The onset of the symmetrical 14 is immediately preceded or accompanied by eye movement to the left. |
62 | Eyes turn right | |
M62 | Eyes right | The onset of the symmetrical 14 is immediately preceded or accompanied by eye movement to the right. |
63 | Eyes up | |
64 | Eyes down | |
65 | Walleye | |
66 | Cross-eye | |
M68 | Upward rolling of eyes | The onset of the symmetrical 14 is immediately preceded or accompanied by an upward rolling of the eyes. |
69 | Eyes positioned to look at other person | The 4, 5, or 7, alone or in combination, occurs while the eye position is fixed on the other person in the conversation. |
M69 | Head and/or eyes look at other person | The onset of the symmetrical 14 or AUs 4, 5, and 7, alone or in combination, is immediately preceded or accompanied by a movement of the eyes or of the head and eyes to look at the other person in the conversation. |
Visibility codes[edit]
AU number | FACS name |
---|---|
70 | Brows and forehead not visible |
71 | Eyes not visible |
72 | Lower face not visible |
73 | Entire face not visible |
74 | Unscorable |
Gross behavior codes[edit]
These codes are reserved for recording information about gross behaviors that may be relevant to the facial actions that are scored.
AU number | FACS name | Muscular basis |
---|---|---|
29 | Jaw thrust | |
30 | Jaw sideways | |
31 | Jaw clencher | masseter |
32 | [Lip] bite | |
33 | [Cheek] blow | |
34 | [Cheek] puff | |
35 | [Cheek] suck | |
36 | [Tongue] bulge | |
37 | Lip wipe | |
38 | Nostril dilator | nasalis (pars alaris) |
39 | Nostril compressor | nasalis (pars transversa) and depressor septi nasi |
40 | Sniff | |
41 | Lid droop | Levator palpebrae superioris (relaxation) |
42 | Slit | Orbicularis oculi muscle |
43 | Eyes closed | Relaxation of Levator palpebrae superioris |
44 | Squint | Corrugator supercilii and orbicularis oculi muscle |
45 | Blink | Relaxation of Levator palpebrae superioris; contraction of orbicularis oculi (pars palpebralis) |
46 | Wink | orbicularis oculi |
50 | Speech | |
80 | Swallow | |
81 | Chewing | |
82 | Shoulder shrug | |
84 | Head shake back and forth | |
85 | Head nod up and down | |
91 | Flash | |
92 | Partial flash | |
97* | Shiver/tremble | |
98* | Fast up-down look |
See also[edit]
References[edit]
- ^Hjortsjö, CH (1969). Man's face and mimic language. free download: Carl-Herman Hjortsjö, Man's face and mimic language'
- ^P. Ekman and W. Friesen. Facial Action Coding System: A Technique for the Measurement of Facial Movement. Consulting Psychologists Press, Palo Alto, 1978.
- ^Paul Ekman, Wallace V. Friesen, and Joseph C. Hager. Facial Action Coding System: The Manual on CD ROM. A Human Face, Salt Lake City, 2002.
- ^ abHamm, J.; Kohler, C. G.; Gur, R. C.; Verma, R. (2011). 'Automated Facial Action Coding System for dynamic analysis of facial expressions in neuropsychiatric disorders'. Journal of Neuroscience Methods. 200 (2): 237–256. doi:10.1016/j.jneumeth.2011.06.023. PMC3402717. PMID21741407.
- ^Freitas-Magalhães, A. (2018). Facial Action Coding System 3.0: Manual of Scientific Codification of the Human Face. Porto: FEELab Science Books. ISBN978-989-8766-86-1.
- ^Freitas-Magalhães, A. (2018).Scientific measurement of the human face:F-M FACS 3.0 - pioneer and revolutionary. In A. Freitas-Magalhães (Ed.), Emotional expression: the Brain and the face (Vol. 10, pp. 21-94). Porto: FEELab Science Books. ISBN978-989-8766-98-4
- ^Freitas-Magalhães, A. (2019). NeuroFACS 3.0: The Neuroscience of Face. Porto: FEELab Science Books. ISBN978-989-8766-61-8.
- ^Freitas-Magalhães, A. (2012). Microexpression and macroexpression. In V. S. Ramachandran (Ed.), Encyclopedia of Human Behavior (Vol. 2, pp. 173–183). Oxford: Elsevier/Academic Press. ISBN978-0-12-375000-6
- ^Del Giudice, M.; Colle, L. (2007). 'Differences between children and adults in the recognition of enjoyment smiles'. Developmental Psychology. 43 (3): 796–803. doi:10.1037/0012-1649.43.3.796. PMID17484588.
- ^Facial Action Coding System. Retrieved July 21, 2007.
- ^Reed, L. I.; Sayette, M. A.; Cohn, J. F. (2007). 'Impact of depression on response to comedy: A dynamic facial coding analysis'. Journal of Abnormal Psychology. 116 (4): 804–809. CiteSeerX10.1.1.307.6950. doi:10.1037/0021-843X.116.4.804. PMID18020726.
- ^Lints-Martindale, A. C.; Hadjistavropoulos, T.; Barber, B.; Gibson, S. J. (2007). 'A Psychophysical Investigation of the Facial Action Coding System as an Index of Pain Variability among Older Adults with and without Alzheimer's Disease'. Pain Medicine. 8 (8): 678–689. doi:10.1111/j.1526-4637.2007.00358.x. PMID18028046.
- ^'Example and web site of one teaching professional: Erika L. Rosenberg, Ph.D'. Archived from the original on 2009-02-06. Retrieved 2009-02-04.
- ^[1]Archived December 23, 2008, at the Wayback Machine
- ^Parr, L. A.; Waller, B. M.; Vick, S. J.; Bard, K. A. (2007). 'Classifying chimpanzee facial expressions using muscle action'. Emotion. 7 (1): 172–181. doi:10.1037/1528-3542.7.1.172. PMC2826116. PMID17352572.
- ^Parr, L. A.; Waller, B. M.; Burrows, A. M.; Gothard, K. M.; Vick, S. J. (2010). 'Brief communication: MaqFACS: A muscle-based facial movement coding system for the rhesus macaque'. American Journal of Physical Anthropology. 143 (4): 625–630. doi:10.1002/ajpa.21401. PMC2988871. PMID20872742.
- ^Waller, B. M.; Lembeck, M.; Kuchenbuch, P.; Burrows, A. M.; Liebal, K. (2012). 'GibbonFACS: A Muscle-Based Facial Movement Coding System for Hylobatids'. International Journal of Primatology. 33 (4): 809. doi:10.1007/s10764-012-9611-6.
- ^Caeiro, C. T. C.; Waller, B. M.; Zimmermann, E.; Burrows, A. M.; Davila-Ross, M. (2012). 'OrangFACS: A Muscle-Based Facial Movement Coding System for Orangutans (Pongo spp.)'. International Journal of Primatology. 34: 115–129. doi:10.1007/s10764-012-9652-x.
- ^Waller, B. M.; Peirce, K.; Caeiro, C. C.; Scheider, L.; Burrows, A. M.; McCune, S.; Kaminski, J. (2013). 'Paedomorphic Facial Expressions Give Dogs a Selective Advantage'. PLoS ONE. 8 (12): e82686. doi:10.1371/journal.pone.0082686. PMC3873274. PMID24386109.
- ^Vick, S. J.; Waller, B. M.; Parr, L. A.; Smith Pasqualini, M. C.; Bard, K. A. (2006). 'A Cross-species Comparison of Facial Morphology and Movement in Humans and Chimpanzees Using the Facial Action Coding System (FACS)'. Journal of Nonverbal Behavior. 31 (1): 1–20. doi:10.1007/s10919-006-0017-z. PMC3008553. PMID21188285.
- ^Friesen, W.; Ekman, P. (1983). EMFACS-7: Emotional Facial Action Coding System. Unpublished manual, University of California, California.
- ^'Facial Action Coding System Affect Interpretation Dictionary (FACSAID)'. Archived from the original on 2011-05-20. Retrieved 2011-02-23.
External links[edit]
- download of Carl-Herman Hjortsjö, Man's face and mimic language' (the original Swedish title of the book is: 'Människans ansikte och mimiska språket'. The correct translation would be: 'Man's face and facial language')
Abstract
Facial expression is widely used to evaluate emotional impairment in neuropsychiatric disorders. Ekman and Friesen’s Facial Action Coding System (FACS) encodes movements of individual facial muscles from distinct momentary changes in facial appearance. Unlike facial expression ratings based on categorization of expressions into prototypical emotions (happiness, sadness, anger, fear, disgust, etc.), FACS can encode ambiguous and subtle expressions, and therefore is potentially more suitable for analyzing the small differences in facial affect. However, FACS rating requires extensive training, and is time consuming and subjective thus prone to bias. To overcome these limitations, we developed an automated FACS based on advanced computer science technology. The system automatically tracks faces in a video, extracts geometric and texture features, and produces temporal profiles of each facial muscle movement. These profiles are quantified to compute frequencies of single and combined Action Units (AUs) in videos, which can facilitate statistical study of large populations in disorders affecting facial expression. We derived quantitative measures of flat and inappropriate facial affect automatically from temporal AU profiles. Applicability of the automated FACS was illustrated in a pilot study, by applying it to data of videos from eight schizophrenia patients and controls. We created temporal AU profiles that provided rich information on the dynamics of facial muscle movements for each subject. The quantitative measures of flatness and inappropriateness showed clear differences between patients and the controls, highlighting their potential in automatic and objective quantification of symptom severity.
1. Introduction
Abnormality in facial expression has been often used to evaluate emotional impairment in neuropsychiatric patients. In particular, inappropriate and flattened facial affect are well-known characteristic symptoms of schizophrenia (; Andreasen (1984a); ; ; ; ; ). Several clinical measures have been used to evaluate these symptoms (Andreasen (1984a,b); ; ). In these assessments, an expert rater codes the facial expressions of a subject using a clinical rating scale such as the SANS (Scale for the Assessment of Negative Symptoms; Andreasen (1984a)), or ratings of positive/negative valence or prototypical categories such as happiness, sadness, anger, fear, disgust and surprise, which are recognized across cultures in facial expressions (Eibl-Eibesfeldt (1970); Ekman and Friesen (1975); ). However, affective impairment from neuropsychiatric conditions often results in 1) ambiguous facial expressions which are combinations of emotions, and 2) subtle expressions which have low intensity or small change, as demonstrated in Figure 1. Consequently, such expressions are difficult to categorize as one of the prototypical emotions by an observer.
Examples of ambiguous (LEFT), subtle (MIDDLE), and inappropriate (RIGHT) facial expressions. The left subject is showing an ambiguous expression, where the upper facial region is showing anger or disgust emotions while the lower facial region is showing a degree of happiness. The middle subject is exhibiting a subtle expression which is barely perceived as sadness by an observer. Additionally, the right subject is displaying an inappropriate expression of happiness when the person is experiencing a disgust emotion. To accurately describe these ambiguous and/or subtle expressions, we use Facial Action Coding System to delineate the movements of individual facial muscle groups.
Ekman and Friesen (1978a,b) proposed the Facial Action Coding System (FACS), which is based on facial muscle change and can characterize facial actions that constitute an expression irrespective of emotion. FACS encodes the movement of specific facial muscles called Action Units (AUs), which reflect distinct momentary changes in facial appearance. In FACS, a human rater can encode facial actions without necessarily inferring the emotional state of a subject, and therefore one can encode ambiguous and subtle facial expressions that are not categorizable into one of the universal emotions. The sensitivity of FACS to subtle expression differences was demonstrated in studies showing its capability to distinguish genuine and fake smiles (), characteristics of painful expressions (; ; ; ; ; ), and depression (). FACS was also used to study how prototypical emotions are expressed as unique combinations of facial muscles in healthy people (Ekman and Friesen (1978a); ; ), and to examine evoked and posed facial expressions in schizophrenia patients and controls (), which revealed substantial differences in the configuration and frequency of AUs in five universal emotions.
Notwithstanding the advantages of FACS for systematic analysis of facial expressions, it has a major limitation. FACS rating requires extensive training, and is time consuming and subjective thus prone to bias. This feature makes investigations on large samples difficult. An automated computerized scoring system is a promising alternative, which aims to produce FACS scores objectively and fast. Our group described computerized measurements of facial expressions with several approaches (; ; ). and quantified regional volumetric difference functions to measure high-dimensional face deformation. These measures were used to classify facial expressions, and produce clinical scores that showed correlations with Video SANS ratings. However, these methods required human operators to manually define regional boundaries and landmarks in face images, which is not suitable for large sample studies. proposed a fully automatic method of analyzing facial expressions in videos by quantifying the probabilistic likelihoods of happiness, sadness, anger, fear, and neutral for each video frame. Case studies with videos of healthy controls and patients with schizophrenia and Asperger's syndrome were reported. However, the method had limited applicability in case of ambiguous or subtle expressions as shown in Figure 1, since it used only four universal emotions.
In this study, we present a state-of-the-art automated FACS system that we developed to analyze dynamic changes of facial actions in videos of neuropsychiatric patients. In contrast to previous computerized methods, the new method 1) analyzes dynamical expression changes through videos instead of still images, 2) measures individual- and combined-facial muscle movements through AUs instead of a few prototypical expressions, 3) performs automatically without requiring interventions from an operator. These advantages facilitate a high-throughput analysis of large sample studies on emotional impairment in neuropsychiatric disorders.
We applied the automated FACS method to videos from eight representative neuropsychiatric patients and controls as illustrative examples to demonstrate its potential applicability to subsequent clinical studies. Qualitative analysis of the videos provides detailed information on the dynamics of the facial muscle movements for each subject, which can aid diagnosis of patients. From the videos, we also computed the frequencies of single and combined AUs for quantitative analysis of differences in facial expressions. Lastly, we used the frequencies to derive measures of flatness and inappropriateness of facial expressions. Flat and inappropriate affect are defining characteristics of abnormalities in facial expression observed in schizophrenia and these measures can serve to quantify these clinical characteristics of neuropsychiatric disorders.
In Section 2, we provide a background review of literature on advances in computerized facial expression recognition systems. In Section 3, we describe the details of our automated FACS system and methods of qualitative and quantitative analysis. In Section 4, we describe pilot data of healthy controls and psychiatric patients, and report the result. We discuss the results and our overall conclusions from the work in Section 5.
2. Background
In this section we briefly summarize advances in the literature on automated facial expression recognition. There is a large volume of computer vision research regarding automatic facial expression recognition. Early work focused on classifying facial expressions in static images into a few prototypical emotions such as happiness, sadness, anger, fear and disgust. However, there has been a growing consensus that recognition of prototypical emotions is insufficient to analyze the range of ambiguous or subtle facial expressions. Consequently, a new line of research focuses on automated rating of facial AUs. Here we review recent work on automatic FACS systems, and refer the reader to Pantic and Rothkrantz (2000b); Fasel and Luettin (2003); Tian et al. (2005); Pantic and Bartlett (2007) for a general review of automated facial expression recognition.
An automated facial action recognition system consists of multiple stages, including face detection/tracking, and feature extraction/classification. Most of the existing approaches have these stages in common, but differ in the exact methods used in each of the stages. We review the literature separately for the two stages.
2.1. Face Detection and Tracking
Automated face analysis, whether for videos or for images, starts with detecting facial regions in the given image frame. Detecting facial regions in an unknown image has been studied intensively in computer vision, and successful methods are known (Viola and Jones (2001); Lienhart and Maydt (2002); Yang et al. (2002)). After determining the face region, further localization of facial components is required to align the faces to account for variations in head pose and inter-subject differences. Several facial action recognition methods bypass exact localization of facial landmarks and use only the centers of eyes and mouth to roughly align the faces, while other methods rely on accurate locations of landmarks. Landmarks are usually localized and tracked with a deformable face model, which matches a pre-trained face model to an unknown face. The likelihood of face appearance is maximized by deforming the model under a learned statistical model of face deformations. Various types of deformable face models have been proposed, including Active Shape Model (ASM: Cootes et al. (1995)), Active Appearance Model (AAM: Cootes et al. (2001)), Constrained Local Model (CLM: Cristinacce and Cootes (2008)), Pictorial Structure (PS: Felzenszwalb and Huttenlocher (2005)), and variations of these models. The geometric deformation determined by landmark locations can be used with a pattern classifier such as Support Vector Machine (Schoelkopf and Smola (2001)) to detect facial actions directly (Lucey et al. (2007); ; ). However, facial actions cause both geometric and texture changes in a face (Ekman and Friesen, (1978b,a)), and therefore more sophisticated methods of feature extraction and classifiers are required for a state-of-the-art system.
2.2. Feature Extraction and Classification
Automated facial action recognition seeks to identify good features from images and videos that capture facial changes, and deploys accurate methods to recognize their complex and nonlinear patterns. Fasel and Luettin (2000) used subspace-based feature extraction followed by nearest neighbor classification to recognize asymmetric facial actions. Lien et al. (2000) used a dense optical flow and high-gradient components as features and a combination of discriminant analysis and Hidden Markov Models (HMM) for recognition. Pantic and Rothkrantz (2000a) and used a detailed parametric description of facial features and Neural Networks to achieve accurate AU recognition with a high-quality database of facial actions (Kanade et al. (2000)). Notably, the process is not fully automated. Pantic and Rothkrantz (2000a) used a sophisticated expert-system to infer the states of individual and combined AUs for still images. Littlewort et al. (2006); Bartlett et al. (2006); Valstar and Pantic (2006) used a large number of Gabor features with Adaboost classifier (Friedman et al. (1998)) to achieve accurate AU recognition, and applied the method to analyze videos. The combination of Gabor features with boosted classifiers proved successful, and our proposed system is built on the framework of these approaches.
Other groups focused on exploiting probabilistic dependency between AUs (Cohen et al. (2003b,a); ; , )). It is observed that certain AUs, for example, Inner Brow Raiser (AU1) and Outer Brow Raiser (AU2), usually accompany each other. Therefore, presence or absence of either AU can help to infer the state of the other AU under ambiguous situations. The probabilistic dependency between AUs was formally modeled by Bayesian Networks, which were learned from observed data. The idea was further extended to handle temporal dependency of AU as well by Dynamic Bayesian Networks. , ) achieved state-of-the-art results for Cohn-Kanade (Kanade et al. (2000)), ISL (), or MMI (Pantic et al. (2005)) databases. However, training a dynamical model requires a large amount of data manually labeled by experts, which could be prohibitive. Dynamical aspects of facial expressions were also emphasized in Yang et al. (2007); , where features for AU were defined from the temporal changes of face appearance. Although a fully dynamical approach has theoretical merits, currently available databases are usually restricted to typical scenarios – posed expressions from a few prototypical emotions or instructed combinations, performed by healthy controls. These scenarios show clear onset, peak, and offset phases. Since dynamics of evoked facial expressions of neuropsychiatric patients are unknown, models learned from a limited training data of healthy controls can be biased and may not be suitable. We therefore do not model such dependency, and classify each AU independently and for each frame.
3. Methods
In this section, we describe the details of the automated FACS system and its application to video analysis. The section is divided into three subsections. In Section 3.1 (Image Processing), we describe how face images are automatically processed for feature extraction. In Section 3.2 (Action Unit Detection), we explain how we train Action Unit classifiers and validate them. In Section 3.3 (Application to Video Analysis), we show how to use the classifiers to analyze videos to obtain qualitative and quantitative information about affective disorders in neuropsychiatric patients.
3.1. Image Processing
3.1.1. Face Detection and Tracking
In the first stage of the automated FACS system, we detect facial regions and track facial landmarks defined on the contours of eyebrows, eyes, nose, lips, etc. in videos. For each frame of the video, an approximate region of a face is detected by the Viola-Jones face detector (Viola and Jones (2001)). The detector is known to work robustly for large inter-subject variations and illumination changes. Within the face region, we search the exact location of facial landmarks using a deformable face model. Amongst the various types of deformable face models, we use Active Shape Model (ASM) for several reasons. ASM is arguably the simplest and fastest method among deformable models, which fit our need to track a large number of frames in multiple videos. Furthermore, ASM is also known to generalize well to new subjects due to its simplicity (Gross et al. (2005)). We train ASM with manually collected 159 landmark locations from a subset of still images that are also used for training AU classifiers. Typical number of landmarks in publicly available databases ranges between 30 and 80. We use 159 landmarks to accurately detect fine movement of facial components (Figure 2). ASM performs better with more landmarks in the model (). When we track landmarks from videos, a face is often occluded by hand or leaves the camera view angle. Such frames are discarded from the analysis automatically. The estimated locations of landmarks for all frames in a video can be further improved by using a temporal model as in . We use a Kalman filter, which combines the observed landmark locations (these are inherently noisy) with the predicted landmarks locations from a temporal model (Wikle and Berliner (2007)). Sample video tracking results are shown in Figure 2. Since the movement of landmarks is also caused by head pose change unrelated to facial expression, it is important to extract the relevant features only as explained in the following sections.
Sample tracking result. On each face, yellow dots indicate the locations of 159 landmarks, and red lines indicate different facial components. Note that accuracy of tracked landmarks is crucial for videos of spontaneously evoked emotions (disgust in this example), since the movement of landmarks due to facial expression is very small compared to the overall movement of the head.
3.1.2. Feature Extraction
We use the detected landmark locations to extract two types of features for AU detection: geometric and texture. Previous facial expression recognition systems typically used either geometric or texture features, not both. We use both features because they convey complementary information. For example, certain AUs can be detected directly from geometric changes: Inner/Outer Brow Raiser (AU1/2) causes displacements of eyebrows, even when the associated texture changes (increased horizontal wrinkles in the forehead) are not visible. However, when Inner Brow Raiser (AU1) is jointly present with Brow Lowerer (AU4), the geometric displacement of eyebrows is less obvious. In that case, texture changes (increased vertical wrinkles between eyebrows) provide complementary evidence of the presence of AU4.
We extract the two features separately and combine them in the classification stage. To define geometric features, we create a landmark template from the training data by Procrustes analysis (Mardia and Dryden (1998)). Starting with the averaged landmark locations as a template, we align landmarks from all training faces to the template, and update the template by averaging the aligned landmarks. This procedure is repeated for a few iterations. From the landmark template, we create a template mesh by Delaunay triangulation, which yields 159 vertices and 436 edges. Deformation of face meshes measured by compression and expansion of the edges reflects facial muscle contraction and relaxation.
To extract geometric features of a test face, we first align the face to the template by similarity transformations to suppress within-subject head pose variations and inter-subject geometric differences. Next, we use differences of edge lengths between each face and a neutral face of the same person, formed into a 436-dimensional vector of geometric features, thereby further emphasizing the change due to facial expressions and suppressing irrelevant changes. Figure 3 demonstrates the procedure for extracting geometric features.
Geometric features for Action Unit Classification. Deformation of the face mesh (LEFT) relative to the mesh at a neutral state (MIDDLE) is computed, and normalized to a subject-independent template mesh (RIGHT). Green and red edges on the template mesh indicate compression and expansion of the edges, respectively.
To extract texture features, we compute a Gabor wavelet response, which has been used widely for face analysis (Pantic and Bartlett (2007)). We use Gabor filter banks with 9 different spatial frequencies from 1/2 to 1/32 in units of pixel−1, and 8 different orientations from 0 to 180 degrees with a 22.5 degree step (Figure 4). Prior to applying the filters, each face image is aligned to a template face using its landmarks, and resized to about 100–120 pixels. The magnitudes of the filters form a 72-dimensional feature at each pixel of the image. In Bartlett et al. (2006), the Gabor responses from the whole image were used as features, whose dimensionality is huge (= 165,888). In Valstar and Pantic (2006), twenty Regions-of-Interest (ROIs) affected by specific AUs were selected. We also used ROIs to reduce the dimensionality, but we took the approach further: Gabor responses in each ROI are pooled by 72-dimensional histograms. This reduces the dimensionality of the features dramatically and makes the features robust to local deformations of faces and errors in the detected landmarks locations. Furthermore, AU classifiers can be trained in much shorter time. The ROIs we use are shown in Figure 5. Similar to geometric features, texture features also have unwanted within-subject and inter-subject variations. For example, a person can have permanent wrinkles in the forehead, which, for a different person, appear only when the eyebrows are raised, and therefore interfere with the correct detection of eyebrow movements. By taking the difference of Gabor response histograms between each face and a neutral face of the same person, we measure the relative change of textures. This approach accounts for newly (dis-)appearing wrinkles as well as deepening of the permanent wrinkles, instead of simple presence or absence of wrinkles.
Examples of Gabor features of multiple spatial frequencies and orientations from a face image. Convolution of an image with Gabor wavelets yields high magnitudes around the structures such as facial contour, eyes and mouth (shown as white blobs in the above images) due to frequency and orientation selectivity of the wavelets. By taking the difference of Gabor features between an emotional face and a neutral face, we can measure the change of facial texture due to facial expressions. Spatial frequency ranges from 1/2 to 1/32 in units of pixel−1, and orientation ranges from 0° to 180° with 22.5° step.
Regions-of-interest (ROIs) for extracting texture features for Action Unit Classification. In each ROI (blue rectangle), a histogram of Gabor features is computed for multiple spatial frequencies and orientations.
3.2. Action Unit Detection
We adopted a classification approach from machine learning to predict the presence or the absence of AUs. A classifier is a general-purpose algorithm that takes features as input and produces a binary decision as output. In our case, we feed a classifier the features extracted from a face (Section 3.1), and the classifier makes a decision whether or not a certain AU is present in the given face. Three necessary steps of a classification approach include 1) data collection, 2) classifier training, and 3) classifier validation, as we describe below.
3.2.1. Collecting Training Data
A classifier ‘learns’ patterns between input features and output decisions from training data, which consist of examples of face images and their associated FACS ratings, from human raters in this case. Our group has been collecting still face images of the universal facial expressions. These included expressions in mild, moderate, and high intensities, in multiple emotions, and in both posed -- subjects were asked to express emotions -- and evoked -- subjects spontaneously expressed emotions – conditions from various demographic groups (, ). Note that the rules of FACS are not affected by these conditions since it only describes the presence of facial muscle movements. These face images were FACS-rated by experts in our groups. There were three initial raters that achieved FACS reliability from the Ekman lab in San Francisco. All subsequent FACS raters had to meet inter-rater reliability of > 0.6 stratified by emotional valence for the presence and absence of all AUs rated on a sample of 128 happy, sad, anger and fear expressions. Two raters - one FACS certified and one FACS reliable - coded presence and absence of AUs in 3419 face images. Instances where ratings differed between the two raters were resolved by visual analysis requiring agreement on absence and presence by both raters. Faces were presented in random order to the raters, along with neutral images of the same person to serve as a baseline face. Among the AUs rated, Lip Tightener (AU23) and Lip Pressor (AU24), which both narrow the appearance of lips, and Lips Part (AU25) and Jaw Drop (AU26), which constitute mouth opening, were collapsed, since they represent differing degrees of the same muscle movement.
3.2.2. Training Classifiers
We selected Gentle Adaboost classifiers (Friedman et al. (1998)) from among a few possible choices of classifiers used in the literature. Adaboost classifiers have several properties that make them preferable to other classifiers for the problem at hand. First, Adaboost selects only a subset of features, which is desirable for handling high-dimensional data. Second, the classifier can adapt to inhomogeneous features (=geometric and texture features) that might have very different distributions. Third, it produces a continuous value of confidence along with its binary decisions through a natural probabilistic interpretation of the algorithm as a logistic regression. We train Adaboost classifiers following Friedman et al. (1998). A total of 15 classifiers are trained to detect each of the 15 AUs independently, using the training data of face images and their associated FACS ratings from human raters. Although the manual FACS ratings included Nasolabial Deepener (AU11), Cheek Pucker (AU13), Dimpler (AU14), and Lower Lip Depressor (AU16), we did not train classifiers for these AUs because the number of positive samples of these AUs in our database was too small to train a classifier reliably.
3.2.3. Validation of Classifiers
Before we used the classifiers, we verified the accuracy of the automated FACS ratings against the human FACS ratings by two-fold cross validation as follows. We divided the training data into two sets. Subsequently, we trained the classifiers with one set using both face images and human ratings, and collected the classifier outputs on the other set using face images only. Then we compared the predicted ratings with the human ratings on the other set. In particular, we divided the training data into posed and evoked conditions to validate that the classifiers are unaffected by these conditions. Table 1 summarizes the agreement rates between automated and manual FACS ratings for 15 AUs representing the most common AUs employed for facial expressions. Overall, we achieved an average agreement of 95.9 %. The high agreement validates the accuracy of the proposed automated FACS.
Table 1
Agreement rates of automated and manual FACS ratings for 15 Action Units
AU No | Description | Rate (%) |
---|---|---|
AU1 | Inner Brow Raiser | 95.8 |
AU2 | Outer Brow Raiser | 97.8 |
AU4 | Brow Lowerer | 91.0 |
AU5 | Upper Lid Raiser | 96.9 |
AU6 | Cheek Raiser | 93.0 |
AU7 | Lid Tightener | 87.0 |
AU9 | Nose Wrinkler | 97.5 |
AU10 | Upper Lip Raiser | 99.3 |
AU12 | Lip Corner Puller | 97.1 |
AU15 | Lip Corner Depressor | 99.2 |
AU17 | Chin Raiser | 96.5 |
AU18 | Lip Puckerer | 98.6 |
AU20 | Lip Stretcher | 97.7 |
AU23 | Lip Tightener | 96.9 |
AU25 | Lips Part | 95.7 |
3.3. Application to Video Analysis
We used the AU classifiers to qualitatively and quantitatively analyze the dynamic facial expression changes in videos. This includes 1) creation of temporal AU profiles, 2) computation of single and combined AU frequencies, and 3) automated measurements of affective flatness and inappropriateness.
3.3.1. Temporal Profiles of AU
The AUs are detected for each and every frame of a video for the whole course of the video, which results in creating temporal AU profiles of the video. Originally, a classifier outputs binary decision (that is, presence or absence of an AU), but it also produces the confidence of the decision (that is, the posterior likelihood of the AU being present) as continuous values in the range of 0 to 1. We use the binary decisions for quantitative analysis and the continuous values for qualitative analysis. When we apply the classifiers to a video, we can create continuous temporal profiles of AUs, which will show the intensity, duration, and timing of simultaneous facial muscle actions in a video.
3.3.2. Analysis of AU Frequencies
Various types of measures can be derived from the AU profiles for quantitative analysis of facial expressions. In , the frequencies of single AUs were analyzed to study group differences between healthy people and schizophrenia patients. AUs were manually rated for a few still images per subject. Our proposed method enables automatic collection of AUs and computation of single AU frequencies for the whole video. We compute:
Frequency of single AUs = number of frames in which an AU is present / total number of frames
Frequency of AU combinations = number of frames in which an AU combination (e.g., AU1+AU4) is present / total number of frames
You can find contactinformation in thesection of these pages.Portions Copyright ©. If worst comes to worst youcould try to contact the manufacturer. Selmer clarinet serial numbers. They may be able to provide you with a list oflicensed qualified appraisers who specialize in different areasie: strings, winds, keyboard, primitive etc.We suggest you contact yourlocal music dealer if you have further questions about purchasingor selling an instrument.Thank You,Leblanc Service Dept.'
The AU combination measures the simultaneous activation of multiple action units in facial expression which is more realistic than isolated movements of single action units, and therefore provides more accurate information than single AU frequencies.
3.3.3. Flatness and Inappropriateness Measure
In the analysis of facial expressions, flatness and inappropriateness of expressions can serve as basic clinical measures for severity of affect expression in neuropsychiatric disorders. For example, in the SANS (Andreasen (1984a)), a psychiatric expert interviews the patients and manually rates the flatness and the inappropriateness of the patient’s affect. However the scales are subjective, require extensive expertise and training, and can vary across raters. By using AU frequencies from the automated FACS method, we can define objective measures of flatness and inappropriateness as follows:
Flatness measure = number of neutral frames in which no AU is present / total number of frames
Inappropriateness measure = number of “inappropriate” frames / total number of frames
To define “inappropriate” frames, we used the statistical study of , which analyzed which AUs are involved in expressing the universal emotions of happiness, sadness, anger, and fear. Specifically, they identified AUs that are uniquely present or absent in each emotion. AUs that are uniquely present in a certain emotion were called “qualifying” AUs of the emotion, and AUs that are uniquely absent were called “disqualifying” AUs of the emotion, as shown in Table 2. Based on this, we defined an image frame from an intended emotion as inappropriate if it contained one or more disqualifying AUs of that emotion or one or more qualifying AUs of the other emotions. This decision rule was applied to all frames in a video to derive the inappropriateness measure automatically.
Table 2
Qualifying and disqualifying Action Units in four emotions (summarized from )
Emotion | Qualifying AUs | Disqualifying AUs |
---|---|---|
Happiness | AU6, AU12 | AU4, AU20 |
Sadness | AU17 | AU25 |
Anger | AU9, AU16 | AU1 |
Fear | AU2 | AU7, AU10 |
4. Results
In this section we describe the acquisition procedure for videos of evoked emotions for pilot data of four healthy controls and four schizophrenia patients representative of variation in race and gender. We apply the qualitative and quantitative analyses developed in Section 3 to these videos and present preliminary results.
4.1. Subjects
Our group has been collecting still images and videos of healthy controls and patients for a neuropsychiatric study of emotions under an approved IRB protocol of the University of Pennsylvania. Participants were recruited from inpatient and outpatient facilities of the University of Pennsylvania Medical Center. After complete description of the study to the subjects, written informed consents, including consent to publish pictures, were obtained. Four healthy controls and four schizophrenia patients were collected for the pilot study. Each group was balanced in gender (two males and two females) and race (two Caucasians and two African-Americans). A summary of the eight subjects and their videos are given in Table 3.
Table 3
Subject | Gender | Race | Qualitative description of the videos |
---|---|---|---|
Control 1 | Male | Caucasian | Mildly expressive, smooth expressions |
Control 2 | Male | African-American | Mildly expressive, lack of defined distinction between emotions |
Control 3 | Female | Caucasian | Expressive, smooth expressions |
Control 4 | Female | African-American | Very expressive, abrupt expressions |
Patient 1 | Male | Caucasian | Very flat, intermittent expressions |
Patient 2 | Male | African-American | Flat, intermittent expressions |
Patient 3 | Female | Caucasian | Mildly expressive, very inappropriate |
Patient 4 | Female | African-American | Very flat, mildly inappropriate |
Facs Ekman
4.2. Acquiring Videos of Evoked Emotions
We followed the behavioral procedure previously described in and subsequently adapted for use in schizophrenia by . Videos were obtained for neutral expressions and for five universal emotions that are reliably rated cross culturally: happiness, sadness, anger, fear, and disgust. Before recording, participants were asked to describe biographical emotional situations, when each emotion was experienced in mild, moderate and high intensities, and these situations were summarized as vignettes. Subsequently, the subjects were seated in a brightly-lit room where recording took place, and these emotional vignettes were recounted to participants in a narrative manner using exact wording derived from the vignettes. The spontaneously evoked facial expressions of the subjects were recorded as videos. Before and between the five emotion sessions, the subjects were asked to relax and return to a neutral state. Each emotion session lasted about 2 minutes.
4.3. Result I: Qualitative Analysis

We applied the AU classifiers to the videos of evoked emotions, which recorded the spontaneous response of the subjects to the recounting of their own experiences. This resulted in continuous temporal profiles of AU likelihoods over the course of the videos. We compared temporal AU profiles of the subjects for five emotions, and show examples which best demonstrate the characteristics of the two groups in Figures 6 – 9. The profiles in Figure 6 represent a healthy control, who exhibited gradual and smooth increase of AU likelihoods and relatively distinct patterns between emotions in terms of the magnitude of common AUs such as Inner Brow Raiser (AU1), Brow Lowerer (AU4), Cheek Raiser (AU6), Lid Tightener (AU7), Lip Corner Puller (AU12), Chin Raiser (AU17), and Lip Puckerer (AU18). The profiles in Figure 7 represent another healthy control, who displayed a very expressive face. Different emotions showed distinctive dynamics. For example, happiness and disgust had several bursts of facial actions, whereas other emotions were more gradual. The profiles in Figure 8 represent a patient, who showed flattened facial action ( that is, mostly a neutral expression) throughout the session, with a few abrupt peaks of individual AUs such as Upper Lid Raiser (AU5), Cheek Raiser (AU6), Lid Tightener (AU7), Chin Raiser (AU17), and Lip Puckerer (AU18). The profiles in Figure 9 represent another patient, which are even flatter than the first patient, except for weak underlying actions of Check Raiser (AU6), Lid Tightener (AU7), and a peak of Brow Lowerer (AU4) in fear. The temporal profiles of other subjects not shown in these figures exhibited intermediate characteristics, that is, they were less expressive than the two control examples but not as flat as the two patient examples.
Temporal Action Units profiles of Control 3 for five emotion sessions. For each Action Unit, the graph indicates the likelihood (between 0 and 1) of the presence of the Action Unit, for the duration of five video (in units of seconds (s)). The subject exhibits gradual and smooth increase of AU likelihoods over time.
Dec 28, 2009 I need a driver for my wheel. Microsoft SideWinder Force Feedback Wheel. The problem is that I cant choose split or combine for the pedals. Some old games that I have, requires this function. Microsoft sidewinder wheel usb drivers. 36 rows Microsoft Sidewinder Precision Racing Wheel Driver for Windows 7 32 bit, Windows 7 64 bit.
Temporal Action Unit profiles of Control 4 for five emotion sessions. The subject exhibits facial actions in most of the AU. There are bursts of facial actions in happiness and disgust, while there are more gradual actions in other emotions.
Temporal Action Unit profiles of Patient 1 for five emotion sessions. The subject exhibits little facial action but for a few abrupt peaks of individual AUs such as AU5, 6, 7, 17, and 18.
Temporal Action Unit profiles of Patient 4 for five emotion sessions. The subject exhibits almost no facial action except for AU4, 6, and 7.
We also compared the temporal profiles for each emotion by selecting a pair of subjects who showed different characteristics. Along with the temporal profiles, captured video frames at 10 randomly chosen time points are selected for visualization with the AU likelihoods indicated by the magnitude of the green bars. Figures 10 – 19 show examples of the temporal profiles of the representative subjects in happiness, sadness, anger, fear, and disgust emotions. In happiness, the first subject (Figure 10) showed gradual increase of Cheek Raiser (AU6), Lid Tightener (AU7), and Lip Corner Puller (AU12), which is typical of a happy expression (), while the second subject (Figure 11) showed little facial action. In sadness, the first subject (Figure 12) showed a convincing sad expression which involved typical AUs such as Lip Corner Depressor (AU15) and Chin Raiser (AU17), while the second subject (Figure 13) showed little facial action. In anger, the first subject (Figure 14) showed a relatively convincing angry face, with an increasing Brow Lowerer (AU4) from 25 (s) to the end, while the second subject (Figure 15) showed fluctuating levels of Cheek Raiser (AU6), Lid Tightener (AU7), and Lip Corner Puller (AU12) between 50 (s) and 90 (s). The expression of the second subject looks far from anger. (More will be discussed in Discussion section with respect to qualifying and disqualifying AUs.) In fear, the first subject (Figure 16) showed flat profiles with little facial action, and the second subject (Figure 17) also showed relatively flat profiles, with a brief period of Cheek Raiser (AU6), Lid Tightener (AU7), and Lip Stretcher (AU20) at around 40 (s), which seems to fail to deliver an expression of fear. In disgust (Figure 18), the first subject showed a convincing disgustful face through the facial actions of Chin Raiser (AU17) and Nose Wrinkler (AU9) along with other AUs, while the second subject (Figure 19) showed relatively flat profiles except for a period of Chin Raiser (AU17) at around 70 (s).
Temporal AU profiles of a subject in happiness emotion. TOP: Captured video frames at several time points (a to j) from the whole duration of the video in units of seconds (s). In each captured video frame, green bars on the right show the same likelihood of action units present. Short bars indicate low or no likelihood and longer bars indicate high likelihood. BOTTOM: The temporal profiles show the likelihood (between 0 and 1) for each Action Unit for the video. Vertical bars indicate the arbitrarily chosen time points (a to j) near the local peaks of Action Units. The subject displays gradual increase of AU6, 7, and 12, which is typical of a happy expression.
Temporal AU profiles of another subject in happiness emotion. The subject displays little facial action.
Temporal AU profiles of a subject in sadness emotion. The subject displays a convincing sad expression which involves typical AUs such as AU15 and 17.
Temporal AU profiles of another subject in sadness emotion. The subject displays little facial action.
Temporal AU profiles of a subject in anger emotion. The subject displays a relatively convincing angry face, with an increasing AU4 from 25 (s) to the end.
Temporal AU profiles of another subject in anger emotion. The subject inappropriately displays fluctuating levels of AU6, 7, and 12 between 50 (s) and 90 (s), which is characteristic of a happy expression.
Temporal AU profiles of a subject in fear emotion. The subject displays little facial action except for AU7.
Temporal AU profiles of another subject in fear emotion. The subject displays little facial action except for a brief period around 40 (s) involving AU6, 7, and 20, which resembles more of a happy expression than fear.
Temporal AU profiles of a subject in disgust emotion. The subject displays a convincing disgustful face through weakly present AU9 and 17 along with other AUs.
Temporal AU profiles of another subject in disgust emotion. The subject displays little facial action except for a period of AU17 at around 70(s)
4.4. Results II: Quantitative Analysis
We computed the single and combined AU frequencies measured from the videos of the eight subjects. We show frequencies from one control and one patient in Tables 4 and and55 as illustrative examples due to space limitation. In single and combined AU frequencies, there are common AUs such as Cheek Raiser (AU6) and Lid Tightener (AU7) that appear frequently across emotions and subjects. However there are many other AUs whose frequencies are different across emotions and subjects. Based on the AU frequencies of all eight subjects, we consequently derived the measures of flatness and inappropriateness (Section 3.3.3) to get more intuitive summary parameters of the AU frequencies. Table 6 summarizes the automated measures for each subject and emotion, except for the inappropriateness of disgust emotion, which was not defined in . The table also shows the flatness and inappropriate measures averaged over all emotions. According to the automated measurement, controls 3, 2, and 4 were very expressive (flatness = 0.0051, 0.0552, 0.1320), while patients 1, 2, and 4 were very flat (flatness = 0.8336, 0.5731, 0.5288). The control 1 and patient 3 were in the medium range (flatness = 0.3848, 0.3076). Inappropriateness of expression was high for patient 4 and 3 (inappropriateness = 0.6725, 0.3398, 0.3150), and was moderate for patient 1, and control 1 – 4 (inappropriateness = 0.2579, 0.2506, 0.2502, 0.1464, 0.0539). The degree of flatness and inappropriateness of expressions varied across emotions, which will be investigated in the future study with a larger population.
Table 4
Frequencies of single AUs from the videos of one control and one patient in different emotions. The frequency of a single AU is defined as the ratio between the number of frames.
Happiness | Sadness | Anger | Fear | Disgust | ||||||
---|---|---|---|---|---|---|---|---|---|---|
Control 1 | AU7 | 1.000 | AU7 | 0.269 | AU7 | 0.905 | AU7 | 0.176 | AU7 | 0.476 |
AU2 | 0.286 | AU4 | 0.154 | AU4 | 0.381 | AU4 | 0.059 | AU6 | 0.381 | |
AU6 | 0.214 | AU6 | 0.381 | AU1 | 0.039 | |||||
AU25 | 0.143 | AU2 | 0.020 | |||||||
Happiness | Sadness | Anger | Fear | Disgust | ||||||
Patient 1 | AU7 | 0.068 | AU1 | 0.049 | AU7 | 0.194 | AU7 | 0.082 | AU17 | 0.071 |
AU5 | 0.045 | AU17 | 0.049 | AU17 | 0.097 | AU5 | 0.041 | AU5 | 0.024 | |
AU18 | 0.023 | AU18 | 0.024 | AU18 | 0.097 | AU6 | 0.041 | |||
AU20 | 0.023 | AU7 | 0.012 | AU5 | 0.016 | AU20 | 0.020 | |||
AU25 | 0.023 |
Table 5
Frequencies of AU combinations from the videos of one control and one patient in different emotions. The combined AU frequency is defined similar to the single AU frequency.
Happiness | Sadness | Anger | Fear | Disgust | ||||||
---|---|---|---|---|---|---|---|---|---|---|
Control 1 | 7 | 0.357 | Neutral | 0.731 | 6+7 | 0.333 | Neutral | 0.765 | Neutral | 0.333 |
2+7 | 0.286 | 4+7 | 0.154 | 4+7 | 0.333 | 7 | 0.137 | 7 | 0.286 | |
6+7 | 0.214 | 7 | 0.115 | 7 | 0.190 | 4+7 | 0.039 | 6 | 0.190 | |
7+25 | 0.143 | Neutral | 0.095 | 4 | 0.020 | 6+7 | 0.190 | |||
4+6+7 | 0.048 | 1 | 0.020 | |||||||
1+2 | 0.020 | |||||||||
Happiness | Sadness | Anger | Fear | Disgust | ||||||
Patient 1 | Neutral | 0.818 | Neutral | 0.890 | Neutral | 0.677 | Neutral | 0.878 | Neutral | 0.905 |
7 | 0.068 | 17 | 0.049 | 7 | 0.129 | 7 | 0.041 | 17 | 0.071 | |
5 | 0.045 | 1 | 0.024 | 18 | 0.065 | 5 | 0.041 | 5 | 0.024 | |
25 | 0.023 | 18 | 0.012 | 7+17 | 0.048 | 6+7 | 0.020 | |||
20 | 0.023 | 1+18 | 0.012 | 17 | 0.032 | 6+7+20 | 0.020 | |||
18 | 0.023 | 1+7 | 0.012 | 17+18 | 0.016 | |||||
7+18 | 0.016 | |||||||||
5 | 0.016 |
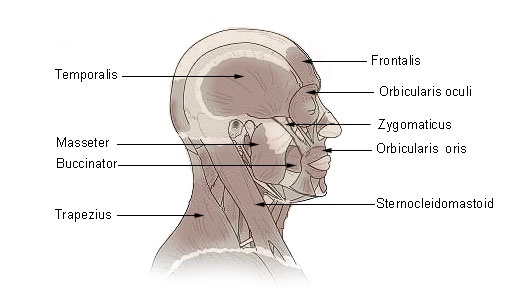
Table 6
Measures of flatness (Flat) and inappropriateness (Inappr) computed from the automated analysis of all eight subjects. Controls 3, 2, and 4 are very expressive (=low flatness), while patients 1, 2, and 4 are very flat. Control 1 and patient 3 are in the medium range. Patients 4 and 3are highly inappropriate, while patient 1 and control 1 – 4 are moderately inappropriate.
Subject | Happiness | Sadness | Anger | Fear | Disgust | Average | ||||||
---|---|---|---|---|---|---|---|---|---|---|---|---|
Flat | Inappr | Flat | Inappr | Flat | Inappr | Flat | Inappr | Flat | Inappr | Flat | Inappr | |
Control 1 | 0.0000 | 0.1739 | 0.7308 | 0.0000 | 0.0952 | 0.2286 | 0.7647 | 0.6000 | 0.7647 | – | 0.3848 | 0.2506 |
Control 2 | 0.0526 | 0.0000 | 0.0000 | 0.1356 | 0.0312 | 0.0857 | 0.1642 | 0.7794 | 0.1642 | – | 0.0552 | 0.2502 |
Control 3 | 0.0000 | 0.0000 | 0.0256 | 0.0714 | 0.0000 | 0.0000 | 0.5143 | 0.0000 | 0.0000 | – | 0.0051 | 0.1464 |
Control 4 | 0.0119 | 0.0470 | 0.2841 | 0.0000 | 0.1224 | 0.0444 | 0.1125 | 0.1240 | 0.1125 | – | 0.1320 | 0.0539 |
Patient 1 | 0.8182 | 0.1250 | 0.8902 | 0.0000 | 0.6774 | 0.2400 | 0.8776 | 0.6667 | 0.8776 | – | 0.8336 | 0.2579 |
Patient 2 | 0.5833 | 0.2286 | 0.3784 | 0.1562 | 0.7805 | 0.2500 | 0.5517 | 0.6250 | 0.5517 | – | 0.5731 | 0.3150 |
Patient 3 | 0.4545 | 0.1190 | 0.1864 | 0.0517 | 0.1869 | 0.3885 | 0.7101 | 0.8000 | 0.7101 | – | 0.3076 | 0.3398 |
Patient 4 | 0.7209 | 0.0000 | 0.6078 | 1.0000 | 0.3036 | 0.8222 | 0.1509 | 0.8679 | 0.1509 | – | 0.5288 | 0.6725 |
5. Discussion
We presented a state-of-the-art method for automated Facial Action Coding System for neuropsychiatric research. By measuring the movements of facial action units, our method can objectively describe subtle and ambiguous facial expressions such as in Figure 1, which is difficult for previous methods that use only prototypical emotions to describe facial expressions. Therefore the proposed system, which uses a combination of responses from different AUs, is more suitable for studying neuropsychiatric patients whose facial expressions are often subtle or ambiguous. While there are other automated AU detectors, they are trained on extreme expressions and hence unsuitable for use in a pathology that manifests as subtle deficits in facial affect expression.
We piloted the applicability of our system in neuropsychiatric research by analyzing videos of four healthy controls and four schizophrenia patients balanced in gender and race. We expect that the temporal profiles of AUs computed from videos of evoked emotions (Figures 6 – 19) can provide clinicians an informative visual summary of the dynamics of facial action. They show which AU or combination of AUs is present in the expressions of an intended emotion from a subject, and quantifies both intensity and duration.
Figures 6 – 9 visualize dynamical characteristics of facial actions of each subject for five emotions at a glance. Overall, these figures revealed that the facial actions of the patients were more flattened compared to the controls. In a healthy control (Figure 6), there was a gradual buildup of emotions that manifests as a relatively smooth increase of multiple AUs. Such a change in profile is expected from the experimental design: the contents of the vignettes progressed from mild to moderate to extreme intensity of emotions. For another control (Figure 7), there were several bursts and underlying activities of multiple AUs. In contrast, patients showed fewer facial actions (Figures 8 and and9),9), and the lack of gradual increase of AU intensities (Figure 8 and and9).9). Also the AU peaks were isolated in time and across AUs (Figure 8). Such sudden movements of facial muscles may be symptomatic of the emotional impairment. These findings lay the basis for a future study to verify the different facial action dynamics patterns in schizophrenia patients and other neuropsychiatric populations.
With the prior knowledge of qualifying and disqualifying AUs for each emotion, we can use the temporal profiles to further aid the diagnosis of affective impairment. Figures 10 and and1111 show the profiles of two subjects in happiness. In the first subject, Cheek Raiser (AU6) and Lip Corner Puller (AU12), which constitute the qualifying AUs of happiness (Table 2), are gradually increasing toward the end of the video, along with Lid Tightener (AU7), which is neither qualifying nor disqualifying. No disqualifying AU is engaged in producing the happiness expression. In contrast, the profiles of the second subject were almost flat. Figures 12 and and1313 show the profiles of two subjects in sadness. With the first subject, the qualifying AU of Chin Raiser (AU17) weakly activated from 30 (s). Although Lip Corner Depressor (AU15) is not a uniquely qualifying AU for sadness, its presence seems to help deliver the sad expression. The profiles of the second subject were flat and showed the weak presence of several AUs (AU6, AU7, AU18, AU23) which are neither qualifying nor disqualifying. Figures 14 and and1515 show the profiles of two subjects in anger. The first subject showed moderate activation of Lid Tightener (AU7) and Brow Lowerer (AU4). These two are neither qualifying nor disqualifying AUs, but they indicate an emotion of a negative valence. The second subject demonstrated an inappropriate expression throughout the duration of the video, which looks closer to happiness than anger. The presence of Cheek Raiser (AU6) and Lip Corner Puller (AU12) in time 50(s) – 100 (s), which constitute the qualifying AUs of happiness, strongly indicates inappropriateness of the expressions, as is Chin Raiser (AU17) at 100 (s) and 200 (s). Figures 16 and and1717 show the profiles of two subjects in fear. The first subject exhibited underlying activity of Cheek Raiser (AU6) and Lid Tightener (AU7) which are inappropriate for fear. The second subject displayed flat expressions except for Cheek Raiser (AU6), Lid Tightener (AU7), Lip Corner Puller (AU12), and Lip Stretcher (AU20) at around 40 (s), which constitute an inappropriate expression for fear. Lastly, Figure 18 and and1919 show the profiles of two subjects in disgust. The first subject was very expressive and showed multiple peaks of Inner Brow Raiser (AU1), Brow Lowerer (AU4), Cheek Raiser (AU6), Lid Tightener (AU7), Nose Wrinkler (AU9), Chin Raiser (AU17), and Lip Tightener (AU23). In contrast, the second subject showed flat profiles except for a brief period of Chin Raiser (AU17) at around 70 (s). We conclude from these findings that the proposed system automatically provides informative summary of the videos to study the affective impairment in schizophrenia, which is much more efficient than manually examining the whole videos by an observer.
The AU profiles from the pilot study were also analyzed quantitatively. From the temporal profiles, we computed the frequency of AUs in each emotion and subject, independently for each AU (Table 4), and jointly for AU combinations (Table 5). AU combinations measure the simultaneous activation of multiple facial muscles, which will shed light on the role of synchronized facial muscle movement in facial expressions of healthy controls and patients. This synchrony cannot be answered by studying single AU frequencies alone. The quantitative measures will allow us to statistically study the differences in facial action patterns between emotions and in multiple demographic or diagnostic groups. Such measures have been used in previous clinical studies (, )) but were limited to a small number of still images instead of videos due to the impractically large amount of effort in rating all individual frames manually.
Lastly, we derived the automated measures of flatness and inappropriateness for each subject and emotion. Table 6 shows that the healthy control has both low flatness and low inappropriateness measures, whereas patients exhibited higher flatness and higher inappropriateness in general. However, there are intersubject variations, for example, control 1 showed a lower inappropriateness but a slightly higher flatnesss than the patient 3. These automated measures of flatness and inappropriateness also agreed with the flatness and inappropriateness from visual examination of the videos (Table 3). The correlation between the automated and the observer-based measurements will be further verified in a future study with a larger sample size. Compared to qualitative analysis, the flatness and inappropriateness measures provide detailed automated numerical information without an intervention of human observers. This highlights the potential of the proposed method to automatically and objectively measure clinical variables of interest, such as flatness and inappropriateness, which can aid in diagnosis of affect expression deficits in neuropsychiatric disorders. It should be noted that while we have acquired the videos with a specific experimental paradigm adopted from former studies, the method we have developed is general and applicable to any experimental paradigm.
The fully automated nature of our method allows us to perform facial expression analysis in large scale clinical study in psychiatric populations. We are currently acquiring a large number of videos of healthy controls and schizophrenia patients for a full clinical analysis. We will apply the method to the data and present the results in a clinical study with detailed clinical interpretation.
Acknowledgment
Funding for analysis and method development was provided by NIMH grant R01-MH073174 (PI: R. Verma); Funding for data acquisition was provided by NIMH grant R01-MH060722 (PI: R. C. Gur).
Footnotes
Facial Action Coding System Manual Pdf
Publisher's Disclaimer: This is a PDF file of an unedited manuscript that has been accepted for publication. As a service to our customers we are providing this early version of the manuscript. The manuscript will undergo copyediting, typesetting, and review of the resulting proof before it is published in its final citable form. Please note that during the production process errors may be discovered which could affect the content, and all legal disclaimers that apply to the journal pertain.
References
Facial Action Coding System Pdf
- Alvino C, Kohler C, Barrett F, Gur R, Gur R, Verma R. Computerized measurement of facial expression of emotions in schizophrenia. J Neurosci Methods. 2007;163(2):350–361.[PMC free article] [PubMed] [Google Scholar]
- Andreasen N. The scale for the assessment of negative symptoms (SANS) 1984a[Google Scholar]
- Andreasen N. The scale for the assessment of positive symptoms (SAPS) 1984b[Google Scholar]
- Bartlett MS, Littlewort G, Frank MG, Lainscsek C, Fasel IR, Movellan JR. Automatic recognition of facial actions in spontaneous expressions. Journal of Multimedia. 2006;1(6):22–35.[Google Scholar]
- Bleuler E. Dementia praecox oder die gruppe der schizophrenien. 1911 [PubMed] [Google Scholar]
- Cohen I, Sebe N, Cozman G, Cirelo M, Huang T. CVPR. 2003a. Learning Bayesian network classifier for facial expression recognition using both labeled and unlabeled data. [Google Scholar]
- Cohen I, Sebe N, Garg A, Chen L, Huang T. Facial expression recognition video sequences: temporal and static modeling. Comput Vis Image Understand. 2003b;91(1–2):160–187.[Google Scholar]
- Cootes TF, Edwards GJ, Taylor CJ. Active appearance models. IEEE Transactions on Pattern Analysis and Machine Intelligence. 2001 Jan;23:681–685.[Google Scholar]
- Cootes TF, Taylor CJ, Cooper DH, Graham J. Active shape models—their training and application. Computer Vision and Image Understanding. 1995;61(1):38–59.[Google Scholar]
- Craig K, Hyde S, Patrick J. Genuine, suppressed and faked facial behavior during exacerbation of chronic low back pain. Pain. 1991;46:161–171. [PubMed] [Google Scholar]
- Cristinacce D, Cootes T. Automatic feature localisation with onstrained local models. Pattern Recognition. 2008 Oct;41(10):3054–3067.[Google Scholar]
- Del Giudice M, Colle L. Differences between children and adults in the recognition of enjoyment smiles. Developmental Psychology. 2007;43(3):796–803. [PubMed] [Google Scholar]
- Eibl-Eibesfeldt I. Ethology, the biology of behavior. New York: Holt, Rinehart and Winston; 1970. [Google Scholar]
- Ekman P, Friesen W. Unmasking the face. Englewood Cliffs: Prentice-Hall; 1975. [Google Scholar]
- Ekman P, Friesen W. Facial Action Coding System: Investigator’s Guide. Palo Altom, CA: Consulting Psychologists Press; 1978a. [Google Scholar]
- Ekman P, Friesen W. Manual of the Facial Action Coding System (FACS) Palo Alto: Consulting Psychologists Press; 1978b. [Google Scholar]
- Fasel B, Luettin J. ICPR. 2000. Recognition of asymmetric facial action unit activities and intensities; pp. 1100–1103. [Google Scholar]
- Fasel B, Luettin J. Automatic facial expression analysis: a survey. Pattern Recognition. 2003;36(1):259–275.[Google Scholar]
- Felzenszwalb PF, Huttenlocher DP. Pictorial structures for object recognition. Int. J. Comput. Vision. 2005;61(1):55–79.[Google Scholar]
- Friedman J, Hastie T, Tibshirani R. Additive logistic regression: a statistical view of boosting. Annals of Statistics. 1998;28:2000.[Google Scholar]
- Gelber E, Kohler C, Bilker W, Gur R, Brensinger C, Siegel S, Gur R. Symptom and demographic profiles in first-episode schizophrenia. Schizophr Res. 2004;67(2–3):185–194. [PubMed] [Google Scholar]
- Gosselin P, Kirouac G, Dor F. Components and Recognition of Facial Expression in the Communication of Emotion by Actors. Oxford: Oxford University Press; 1995. pp. 243–267. [PubMed] [Google Scholar]
- Gross R, Matthews I, Baker S. Generic vs. person specific active appearance models. Image Vision Comput. 2005 Nov;23(1):1080–1093.[Google Scholar]
- Gur R, Kohler C, JD R, SJ S, Lesko K, Bilker W, Gur R. Flat affect in schizophrenia: Relation to emotion processing and neurocognitive measures. Schizophr Bull. 2006;32(2):279–287.[PMC free article] [PubMed] [Google Scholar]
- Gur R, Sara R, Hagendoorn M, Marom O, Hughett P, Macy L, Turner T, Bajcsy R, Posner A, Gur R. A method for obtaining 3-dimensional facial expressions and its standardization for use in neurocognitive studies. J. Neurosci. Methods. 2002;115(2):137–143. [PubMed] [Google Scholar]
- Izard C. Innate and universal facial expressions: evidence from developmental and cross-cultural research. Psychol. Bull. 1994;115(2):288–299. [PubMed] [Google Scholar]
- Kanade T, Tian Y, Cohn JF. Comprehensive database for facial expression analysis. Automatic Face and Gesture Recognition. 2000;0:46.[Google Scholar]
- Koelstra S, Pantic M, Patras IY. A dynamic texture-based approach to recognition of facial actions and their temporal models. IEEE Trans. Pattern Anal. Mach. Intell. 2010;32:1940–1954. [PubMed] [Google Scholar]
- Kohler C, Gur R, Swanson C, Petty R, Gur R. Depression in schizophrenia: I. association with neuropsychological deficits. Biol. Psychiatry. 1998;43(3):165–172. [PubMed] [Google Scholar]
- Kohler C, Turner T, Stolar N, Bilker W, Brensinger C, Gur R, Gur R. Differences in facial expressions of four universal emotions. Psychiatry Res. 2004;128(3):235–244. [PubMed] [Google Scholar]
- Kohler CG, Martin EA, Stolar N, Barrett FS, Verma R, Brensinger C, Bilker W, Gur RE, Gur RC. Static posed and evoked facial expressions of emotions in schizophrenia. Schizophr. Res. 2008;105[PMC free article] [PubMed] [Google Scholar]
- Kotsia I, Pitas I. Facial expression recognition in image sequences using geometric deformation features and support vector machines. IEEE Trans. Image Process. 2007;16(1):172–187. [PubMed] [Google Scholar]
- Kring A, Kerr S, Smith D, Neale J. Flat affect in schizophrenia does not reflect diminished subjective experience of emotion. J. Abnorm. Psychol. 1993;102(4):507–517. [PubMed] [Google Scholar]
- Kring A, Sloan D. The facial expression coding system (faces): development, validation, and utility. Psychol. Assess. 2007;19(2):210–224. [PubMed] [Google Scholar]
- Larochette A, Chambers C, Craig K. Genuine, suppressed and faked facial expressions of pain in children. Pain. 2006;126:64–71. [PubMed] [Google Scholar]
- Lien J, Kanade T, Cohn J, Li C-C. Detection, tracking, and classification of action units in facial expression. Robotics Autonomous Syst. 2000;31:131–146.[Google Scholar]
- Lienhart R, Maydt J. An extended set of haar-like features for rapid object detection. IEEE Conf. ICIP. 2002:900–903.[Google Scholar]
- Lints-Martindale A, Hadjistavropoulos T, Barber B, Gibson S. A psychophysical investigation of the facial action coding system as an index of pain variability among older adults with and without Alzheimer’s disease. Pain Medicine. 2007;8(8) [PubMed] [Google Scholar]
- Littlewort G, Bartlett MS, Fasel IR, Susskind J, Movellan JR. Dynamics of facial expression extracted automatically from video. Image Vision Comput. 2006;24(6):615–625.[Google Scholar]
- Lucey S, Ashraf AB, Cohn J. Investigating spontaneous facial action recognition through AAM representations of the face. In: Kurihara K, editor. Face Recognition Book. Mammendorf, Germany: Pro Literatur Verlag; 2007. Apr, [Google Scholar]
- Mardia KV, Dryden IL. Statistical Shape Analysis. Chichester: Wiley; 1998. [Google Scholar]
- Pantic M, Bartlett M. Machine Analysis of Facial Expressions. Vienna, Austria: I-Tech Education and Publishing; 2007. pp. 377–416. [Google Scholar]
- Pantic M, Rothkrantz L. Expert system for automatic analysis of facial expressions. Image Vision Comput. 2000a Aug;18(11):881–905.[Google Scholar]
- Pantic M, Rothkrantz LJ. Automatic analysis of facial expressions: The state of the art. IEEE Trans. Pattern Anal. Mach. Intell. 2000b;22:1424–1445.[Google Scholar]
- Pantic M, Valstar M, Rademaker R, Maat L. Web-based database for facial expression analysis. IEEE Conf. Multimedia and Expo. 2005[Google Scholar]
- Prkachin K. The consistency of facial expressions of pain: a comparison across modalities. Pain. 1992;58:297–306. [PubMed] [Google Scholar]
- Prkachin K, Mercer S. Pain expression in patients with shoulder pathology: validity, properties and relationship to sickness impact. Pain. 1989;39:257–265. [PubMed] [Google Scholar]
- Reed L, Sayette M, Cohn J. Impact of depression on response to comedy: A dynamic facial coding analysis. J Abnormal Psychology. 2007;116(4):804–809. [PubMed] [Google Scholar]
- Rocha E, Prkachin K, Beaumont S, Hardy C, Zumbo B. Pain reactivity and somatization in kindergarten-age children. J Pediatr Psychol. 2003;28:47–57. [PubMed] [Google Scholar]
- Schoelkopf B, Smola AJ. Learning with Kernels: Support Vector Machines, Regularization, Optimization, and Beyond. Cambridge, MA, USA: MIT Press; 2001. [Google Scholar]
- Shtasel D, Gur R, Gallacher F, Heimberg C, Gur R. Gender differences in the clinical expression of schizophrenia. Schizophr. Res. 1992;7(3):225–231. [PubMed] [Google Scholar]
- Stegmann M, Ersbol B, Larsen R. Famea flexible appearance modeling environment. IEEE Trans Med Imaging. 2003;22(10):1319–1431. [PubMed] [Google Scholar]
- Tian Y-L, Kanade T, Cohn J. Handbook of face recognition. Springer; 2005. [Google Scholar]
- Tian Y-l, Kanade T, Cohn JF. Recognizing action units for facial expression analysis. IEEE Trans. Pattern Anal. Mach. Intell. 2001;23(2):97–115.[PMC free article] [PubMed] [Google Scholar]
- Tong Y, Chen J, Ji Q. A unified probabilistic framework for spontaneous facial action modeling and understanding. IEEE Trans. Pattern Anal. Mach. Intell. 2010;32:258–273. [PubMed] [Google Scholar]
- Tong Y, Liao W, Ji Q. Facial action unit recognition by exploiting their dynamic and semantic relationships. IEEE Trans. Pattern Anal. Mach. Intell. 2007;29(10):1683–1699. [PubMed] [Google Scholar]
- Valstar M, Pantic M. Fully automatic facial action unit detection and temporal analysis. IEEE Conf. CVPR. 2006:149.[Google Scholar]
- Verma R, Davatzikos C, Loughead J, Indersmitten T, Hu R, Kohler C, Gur R, Gur R. Quantification of facial expressions using high-dimensional shape transformations. J. Neurosci. Methods. 2005;141(1):61–73. [PubMed] [Google Scholar]
- Viola P, Jones M. Rapid object detection using a boosted cascade of simple features. IEEE Conf. CVPR. 2001:511–518.[Google Scholar]
- Walker E, Grimes K, Davis D, Smith A. Childhood precursors of schizophrenia: facial expressions of emotion. Am. J. Psychiatry. 1993;150(11):1654–1660. [PubMed] [Google Scholar]
- Wang P, Barrett F, Martin E, Milonova M, Gur RE, Gur RC, Kohler C, Verma R. Automated video-based facial expression analysis of neuropsychiatric disorders. J. Neurosci. Methods. 2008;168:224–238.[PMC free article] [PubMed] [Google Scholar]
- Wikle C, Berliner L. A bayesian tutorial for data assimilation. Physica D. 2007;230(1–2):1–16.[Google Scholar]
- Yang M-H, Kriegman D, Ahuja N. Detecting faces in images: a survey. IEEE Trans. Pattern Anal. Mach. Intell. 2002;24(1):34–58.[Google Scholar]
- Yang P, Liu Q, Metaxas DN. Boosting coded dynamic features for facial action units and facial expression recognition. IEEE Conf. CVPR. 2007:1–6.[Google Scholar]
- Zhang Y, Ji Q. Active and dynamic information fusion for facial expression understanding from image sequences. IEEE Trans. Pattern Anal. Mach. Intell. 2005 May;27:699–714. [PubMed] [Google Scholar]